Hyper Luminal is an indie games studio based in Dundee, Scotland. Everything we make is inspired by our ambition to build high-quality games that create lasting memories and fill our team with pride.
Games
Hyper Luminal is creative, ambitious, and is making its mark on the industry with unique, high-quality games that fill our team with pride and create lasting memories. We make games where every team member proudly states: “I built that!”. We put smiles on people’s faces with beautiful and fun experiences that people play and love.

Work for Hire
Hyper Luminal has delivered 70+ games by working with a wide spectrum of clients, from high profile entertainment brands to other independent games developers. We take an active role in all aspects of the development pipeline including; Concept, Design, Core Production, Quality Assurance, Porting and LiveOps.

About Us
The studio was founded in Dundee, Scotland; a place historically known for its game industry talent. Dundee plays host to a large, close-knit community of independent games developers and is home to the world-class games institution: Abertay University. The city is the root of our knowledge and learning; it’s where creativity thrives and we have become an important part of Dundee’s gaming history.

Associations
Sustainability is a key pillar, that’s why we continue to ensure we are at the forefront of initiatives that improve our studio. We partner with NetXero on our carbon footprint. We protect our team with the latest cyber security measures, and of course, we ensure our entire team is paid the National Living Wage.

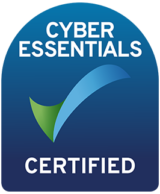
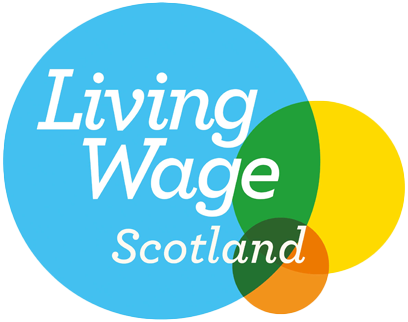
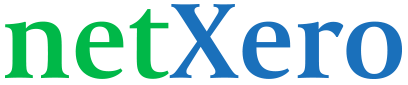